AI learns to predict mental health diagnoses, results can be used for prevention
Artificial intelligence learned to identify traits that can predict a future mental health diagnosis from responses to an occupational health questionnaire assessing health and wellbeing, according to a study by Terveystalo and the Finnish Institute of Occupational Health. The finding sheds light on the potential of AI in identifying and preventing mental health problems.
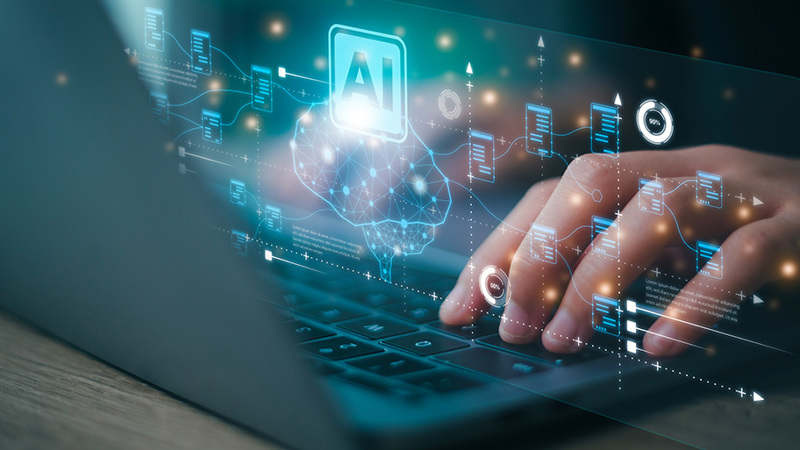
The National Institute of Occupational Health, together with Terveystalo, carried out a study in which artificial intelligence searched for factors predictive of mental health diagnosis from health questionnaire responses.
– The use of AI and machine learning can make healthcare processes more efficient, faster and more accurate in the future. In the future, we will be able to do complex things without human hands, which will bring significant benefits in terms of time allocation for healthcare professionals. The development of algorithms needs to be done responsibly, and regulation in this area is not yet fully complete," said Simo Taimela, senior epidemiologist at Terveystalo, who was involved in the study.
Mental health disorders account for a significant proportion of sick leave: they are also the main cause of disability in Finland
Mental health problems are currently the biggest cause of longer sick leave and disability in Finland. Early identification and prevention of mental health problems is increasingly important for working life.
– New approaches offered by data-driven approaches can increase the quality and effectiveness of care. By predicting the risks of illness, we can intervene proactively to prevent risk factors, prevent the onset of illness and thus reduce mental health-related sickness absence and disability. Anticipating the need for treatment also helps to better allocate specialist resources, which can in turn ease the treatment gap," adds Taimela.
Predictors of mental health diagnosis included factors related to mood and sleep, and study revealed gender differences
In the study, questions about feeling stressed, daytime fatigue and sadness were the most predictive of a future mental health diagnosis. The AI also recognised that age and gender matter.
– For example, the experience of a hopeless future and income from work may predict a future mental health diagnosis more in men than in women. For women, work-life balance also emerged as one of the most important factors. Among physical symptoms, headaches and poor sleep played a role," says Taimela.
Early treatment of mental challenges is important
Daytime fatigue, which emerged as one of the predictive signals in the study, can be caused by sleep problems or a lack of adequate recovery.
– If you are experiencing daytime fatigue or sadness, getting preventive support quickly is important. Help can be sought through low-threshold discussion channels or from an occupational health psychologist, says Sari Nuikki, senior occupational health psychologist at Terveystalo.
It is important to treat all mental health challenges early, not after symptoms have become prolonged or chronic. For example, brief psychotherapy in occupational health has been shown to be effective in preventing mental health absenteeism and diagnoses.
– Fortunately, the stigma around mental health is receding and people are daring to talk about it more openly. For example, the number of visits to Terveystalo for anxiety and depression diagnoses has increased by about a third in the 2020s," says Nuikki.
Employers are now also more strongly expected to recognise and manage mental stress
In the June update of the Occupational Safety and Health Act, employers' obligations were clarified and they are now also more strongly required to identify and manage psychosocial stress. Here, too, AI data can help in the future.
Front-line workers, as the employer's representative, have a key role to play in identifying and preventing harmful stress.
– Harmful psychosocial stress can be caused, for example, by excessive data volumes, constant interruptions, difficult customer situations, excessive workloads and dysfunctions in the functioning of the work community. When we detect early warning signs of mental health problems, it is important to intervene as early as possible to avoid deepening problems," says Nuikki.
The study in brief
- The data for the study consisted of occupational health questionnaires and mental health diagnoses from 11,828 occupational health clients of Terveystalo.
- Those with a diagnosis before completing the questionnaire were removed and the follow-up period from completing the occupational health questionnaire to a possible mental health diagnosis was limited to two years.
- Guided machine learning was used to classify the data.
A classifier trained with questionnaire responses was clearly better than a random classifier, but questionnaire responses alone cannot yet predict mental health diagnoses in individual cases with complete confidence. - Managing and supporting well-being at work requires skilled and adequately resourced people to do the everyday things that support workers' well-being.
Read more occupational health articles
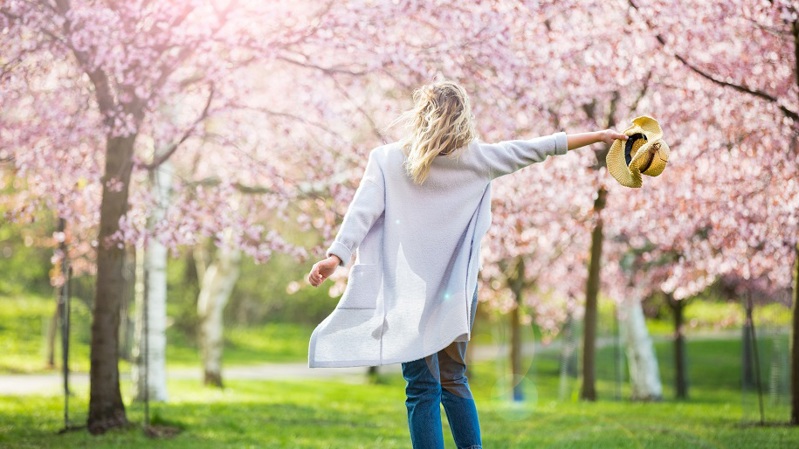
A mind-friendly and brain-healthy holiday - how to recover and recharge your batteries?
Many people start their summer holidays after Midsummer. For a holiday to soothe your mind, take a look at Terveystalo's occupational health psychologist Tiina Tuominen's tips for a restorative holiday.
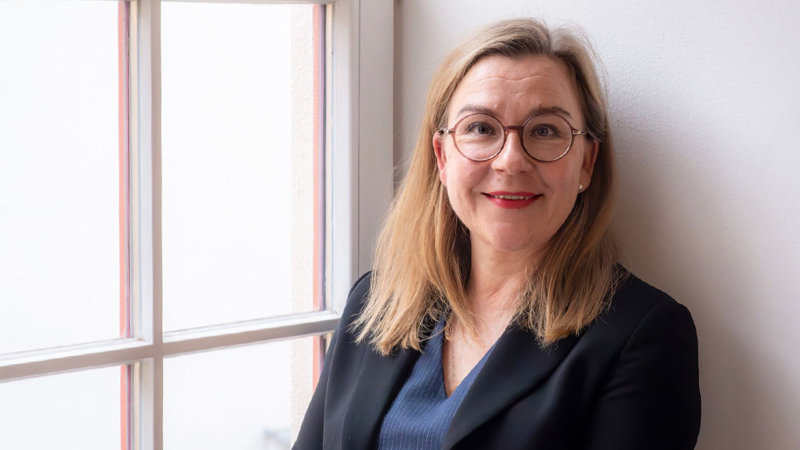
What future leadership looks like?
According to Hertta Vuorenmaa, an expert on the future of work and management, work is a huge social institution, and changing it is always difficult because we have established ideas about how to do work. Understanding historical turning points can help in managing current changes, as changes in working life are not only technology-driven, but require holistic thinking and managing people's emotions.
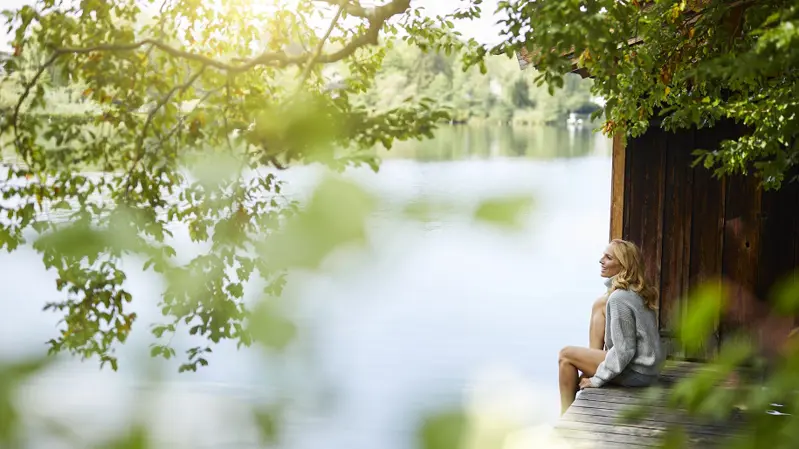
The approaching holiday feels stressful – What can help?
The holiday is approaching, but work issues are piling up and there’s conflict in the family about how to spend the time off. Excessive expectations and the pressure to “make the most of it” can cause unnecessary anxiety.
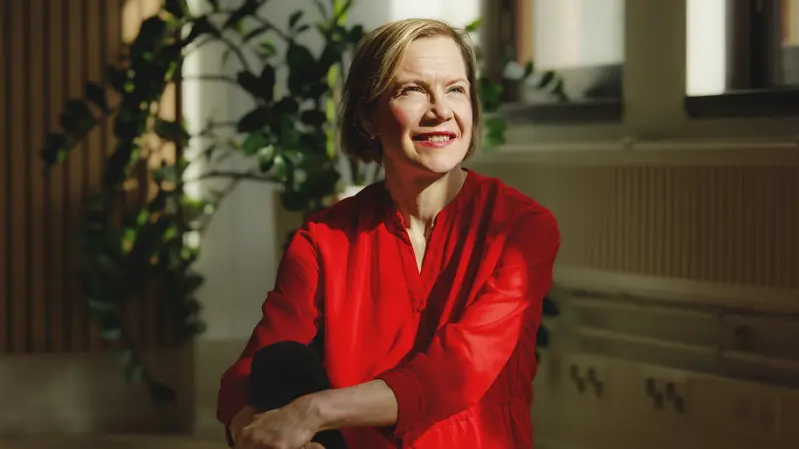
"Let life show at work"
Humanity in the workplace feeds well-being and productivity. Workplaces need a new culture that genuinely values diversity and takes into account different life situations," says Eveliina Holmgren, an organisational psychologist at Terveystalo.
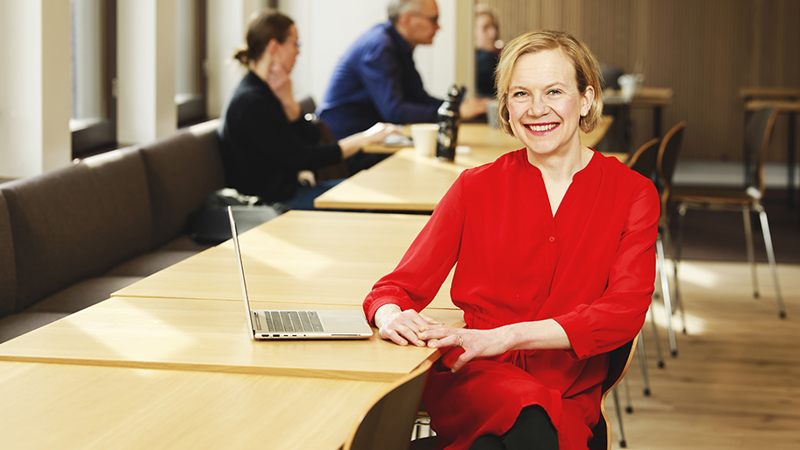
Tips from an organisational psychologist: how to create psychological safety in the workplace
Eveliina Holmgren, an organisational psychologist at Terveystalo, explains how to get started in strengthening psychological safety in the workplace.
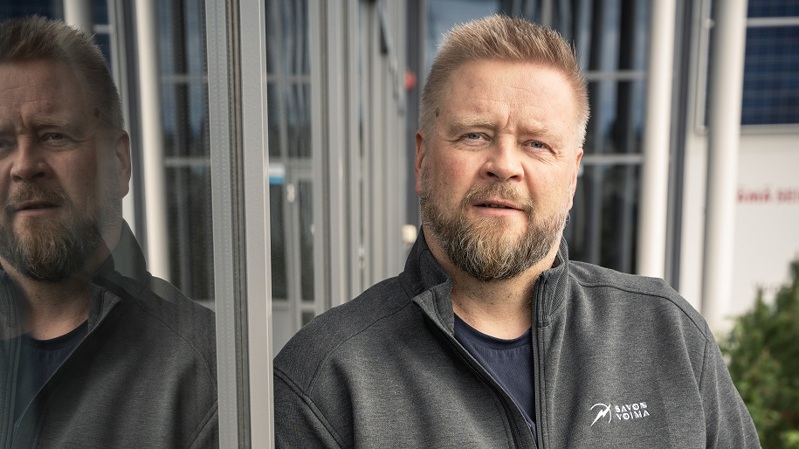
Get rid of type 2 diabetes with lifestyle changes
When Timo Partanen found out at his health check that he had type 2 diabetes, he realised that he would end up taking medication for the rest of his life if things continued as they were. The diagnosis made him think about his future and motivated him to make a permanent change.